Morges, Switzerland, 10th of January
Evgeny Bogdanov– GAMAYA, CTO.
As AI continues to penetrate every industry, agriculture is undergoing a similar transformation. At Gamaya, we are leading this technological revolution, utilizing advanced AI techniques to drive growth and efficiency in the sugarcane sector worldwide.
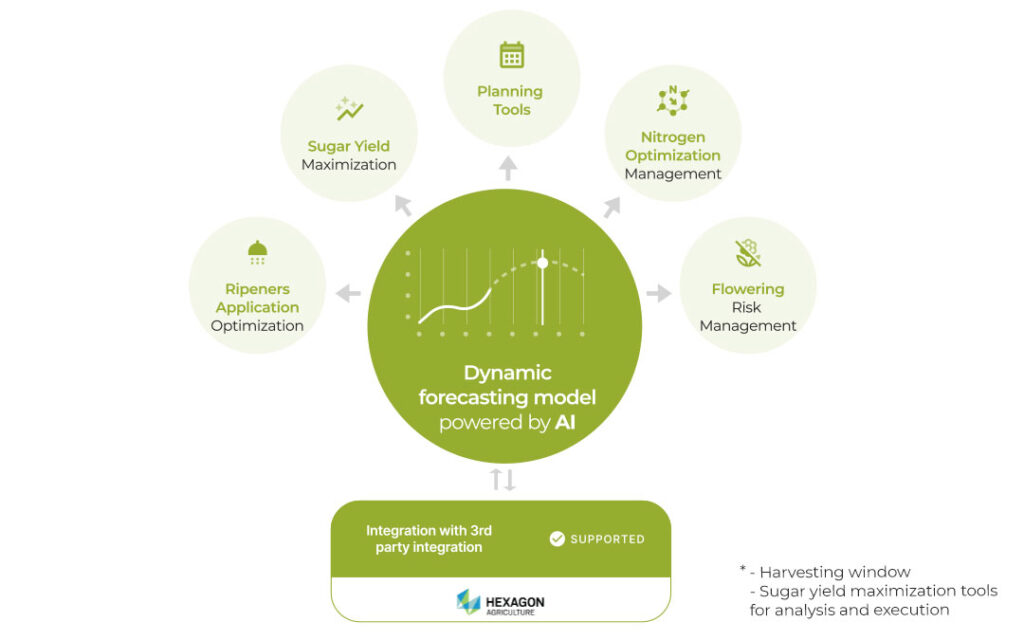
Our core engine for sugarcane growers is the forecasting model, which predicts crop development throughout the full vegetation cycle. This capability is essential for sugarcane mills, as accurate forecasting enables them to make key operational decisions, including budget planning, commercial decisions, optimizing harvesting operations, preventing biological risks, and optimizing fertilizer applications. Accurate forecasting is directly linked to sugar or ethanol production, the primary KPIs in the sugarcane industry.
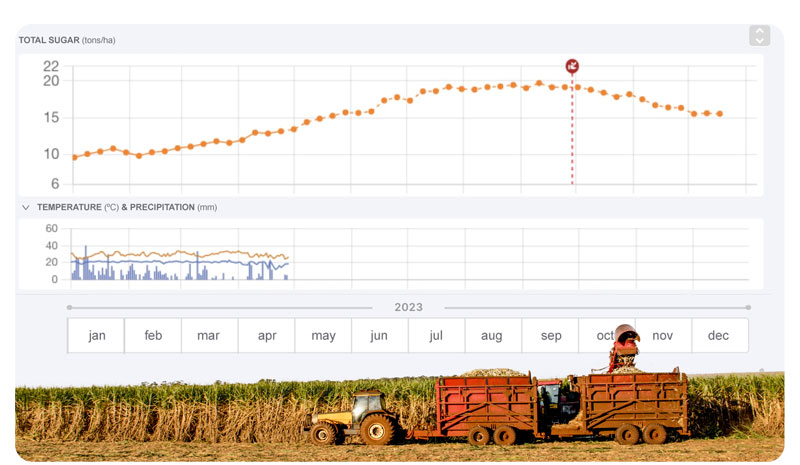
Our data science models estimate biomass yield and sugar content for any time point in the future on a weekly basis, enabling mills to make the best operational decisions to improve business performance and reduce sugar content.
Classical Machine Learning journey: good but not great
Initially, our predictive models for sugarcane Yield and Sugar content were developed using classical machine learning approaches. While our drone analytics already utilized deep learning methodologies, our Yield and Sugar content predictive models were still in their early stages, with data acquisition ongoing throughout 2022. To train our models, we use inputs such as weather, remote sensing data, agronomic field attributes (variety, planting date, etc.), and soil type. These inputs are then tuned using historical data from multiple years to provide the most accurate predictions possible.
Our data scientists conducted a process of feature engineering, analyzing various data sources to build a list of input features that have the best correlation with Sugar content and Yield. The features are often inspired by agronomic knowledge, and multiple experiments are conducted to determine which features are most important for better predictions. For example, we discovered that the area of the NDVI growth curve highly correlates with sugarcane Yield (biomass), leading to ndvi-area becoming one of the features used in our model prediction. Overall, we utilize around 150 input features to train our models.
While classical machine learning with feature engineering has its benefits, it also has limitations and issues. While our classical ML models provided good results, we quickly encountered blockers where the model overestimated the forecast due to the way input features were engineered. For instance, we noticed that the predictions in the middle of the season were several percentages higher than the targets, an effect not present towards the end of the season. These issues led us to experiment with deep learning techniques to further improve the accuracy of our models.
Deep learning methods raising the bar of the accuracy
By the time we began exploring deep learning approaches, we had already amassed a significant amount of training data. Deep learning not only helped us resolve the overestimation problem but also dramatically improved overall model performance. Our transition from classical machine learning to deep learning has allowed us to further enhance our forecasting capabilities, ultimately leading to better support for sugarcane mills and the agriculture industry as a whole.
And this is how this works
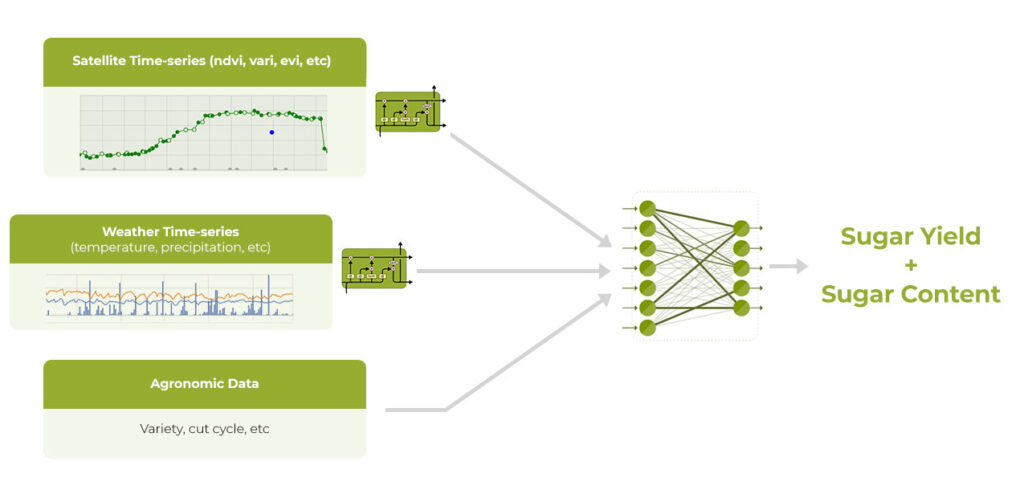
To measure the performance of our yield and sugar content predictive models, we use two metrics: mill level error and field level error.
The mill level error is the average amount of biomass (ton/ha) for the entire mill, which is a highly precise number directly related to the total biomass harvested by a sugarcane mill. The field level error is the average relative error measured across each individual sugarcane field. This number may contain errors due to manual errors or missing data recorded during harvesting operations.
Our deep learning approach has enabled us to improve the accuracy of our yield and sugar content predictive models significantly. We have achieved exceptional accuracy metrics of up to 94% at the single parcel level and up to 98% at the full farm level.
Moreover, our new deep learning model has resolved the overestimation issue, which was a significant problem with our classical machine learning approach, leading to an improvement in overall accuracy. However, we believe we can further enhance the performance of our models by leveraging new data sources, such as higher resolution and more frequent weather and satellite imagery, new sensors, and more historical data.
Our team is currently experimenting with these data sources to improve the precision of our models, which will better handle cross-seasonal climate variations.
Additionally, we are working on extrapolating our modelling experience to model changes in soil organic content, which is essential for verifying carbon removal programs in the sugarcane sector.
At Gamaya, we are committed to revolutionising the agriculture industry by developing and utilising cutting-edge technology to drive growth and efficiency in sugarcane production. Our predictive analytics models for sugarcane Yield and Sugar content are just one of the many ways in which we are accomplishing this goal.